Data poisoning: How Artists Are Sabotaging AI To Take Revenge On Image Generators.
You enter the prompt: “red balloon against a blue sky” but the generator returns an image of an egg instead. You try again but this time, the generator shows an image of a watermelon.
Faheem Hassan
12/18/20233 min read
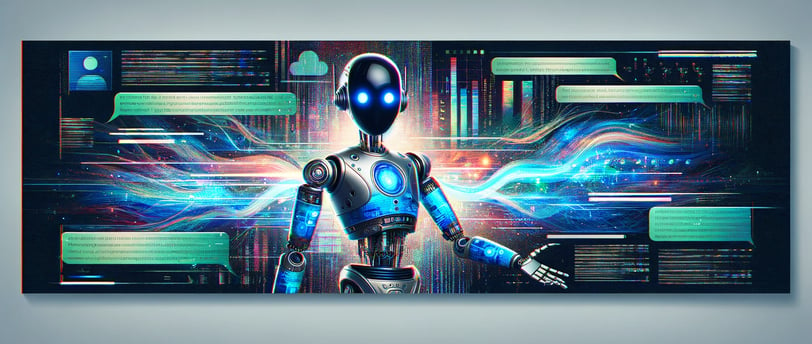
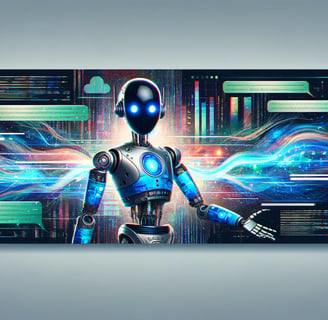
ElevenLabs.io is a great platform for generating realistic and expressive voices from text.
What is 'Data Poisoning'?
Text-to-image generators operate by training on extensive datasets containing millions or even billions of images. Some generators, like those developed by Adobe or Getty, restrict their training to images they own or for which they have a license.
However, other generators are trained using a wide net of online images, many potentially under copyright. This practice has led to numerous copyright infringement lawsuits, with artists accusing major tech firms of using and profiting from their work without permission.
This situation gives rise to the concept of "poisoning." To support individual artists, researchers have recently developed a tool called "Nightshade," designed to combat unauthorized image scraping.
Nightshade functions by subtly modifying an image's pixels in a manner that disrupts computer vision algorithms while remaining imperceptible to human eyes.
If a company then uses one of these altered images for training a future AI model, the integrity of their data is compromised, or "poisoned." This can lead the algorithm to incorrectly categorize images in ways that are visibly incorrect to humans, causing the generator to produce erratic and unintended outcomes.
Indications of Data Poisoning For instance, a balloon might be misclassified as an egg. A request for a Monet-style image could erroneously produce a Picasso-style image.
Previous AI model issues, such as difficulties in accurately rendering hands, might reemerge. Additionally, these models might start incorporating bizarre and irrational elements into images – like six-legged dogs or misshapen sofas.
The more "poisoned" images there are in the training dataset, the more pronounced the disruption. Due to the nature of generative AI, the impact of these "poisoned" images extends to related search keywords as well.
For instance, if training data includes a "poisoned" image of a Ferrari, this can skew the results for prompts related to other car brands or terms like 'vehicle' and 'automobile'.
The creator of Nightshade aims for this tool to encourage major tech companies to respect copyright laws. However, there's a risk that individuals might misuse Nightshade, uploading "poisoned" images to generators to intentionally disrupt their services.
Is There a Solution? To counteract this, various stakeholders have suggested a mix of technological and manual solutions. A key strategy is to scrutinize the sources of input data and its permissible uses, thereby reducing random data collection.
This method, however, challenges a prevalent assumption among computer scientists: the belief that data available online is fair game for any purpose.
Other technical solutions include "ensemble modeling," which involves training different models on varied data subsets and comparing them to identify anomalies. This method is useful not only for training but also in detecting and eliminating potential "poisoned" images.
Auditing is another viable option. One auditing method is to develop a "test battery" – a small, meticulously curated, and well-labeled dataset using "hold-out" data never involved in training. This set serves as a benchmark to test the model's accuracy.
Countering Technological Challenges "Adversarial approaches," like data poisoning, which aim to degrade or deceive AI systems, are not new concepts. Historically, these have included using makeup and costumes to evade facial recognition technologies.
Human rights advocates have long been concerned about the unregulated use of machine vision, especially regarding facial recognition.
For example, Clearview AI, with its extensive, searchable facial database sourced from the internet, is utilized by law enforcement and governments globally. In 2021, the Australian government concluded that Clearview AI violated the privacy rights of its citizens.
In reaction to the use of facial recognition systems for profiling individuals, including legitimate protesters, artists have developed adversarial makeup designs. These patterns, characterized by jagged lines and asymmetric curves, are effective in preventing surveillance systems from accurately identifying individuals.
This scenario is closely linked to the concept of data poisoning, as both situations pertain to broader concerns about the governance and ethical use of technology.
While many technology providers might view data poisoning as a mere nuisance to be resolved through technical means, it might be more appropriate to regard data poisoning as a creative response to the infringement on the basic moral rights of artists and users. This perspective challenges the conventional approach to technological development and emphasizes the importance of ethical considerations in the digital realm.